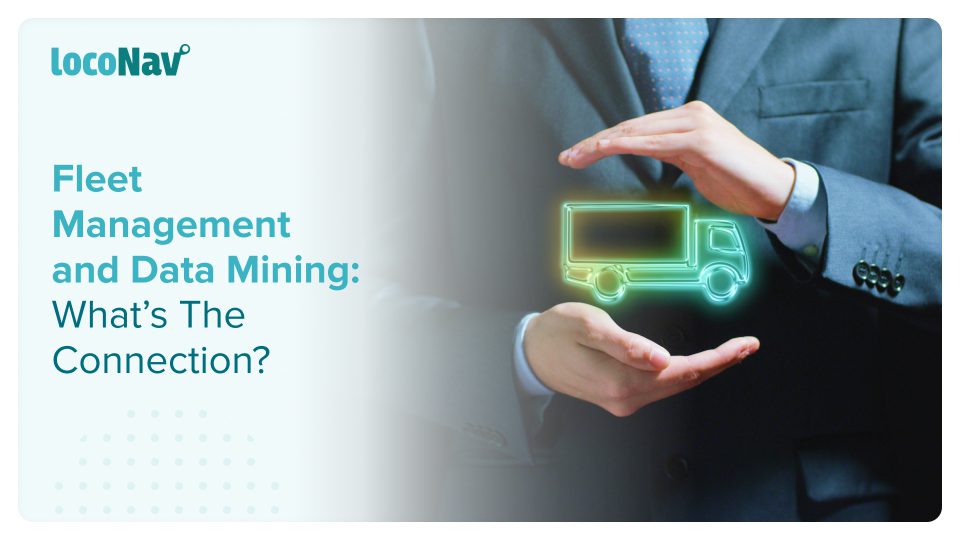
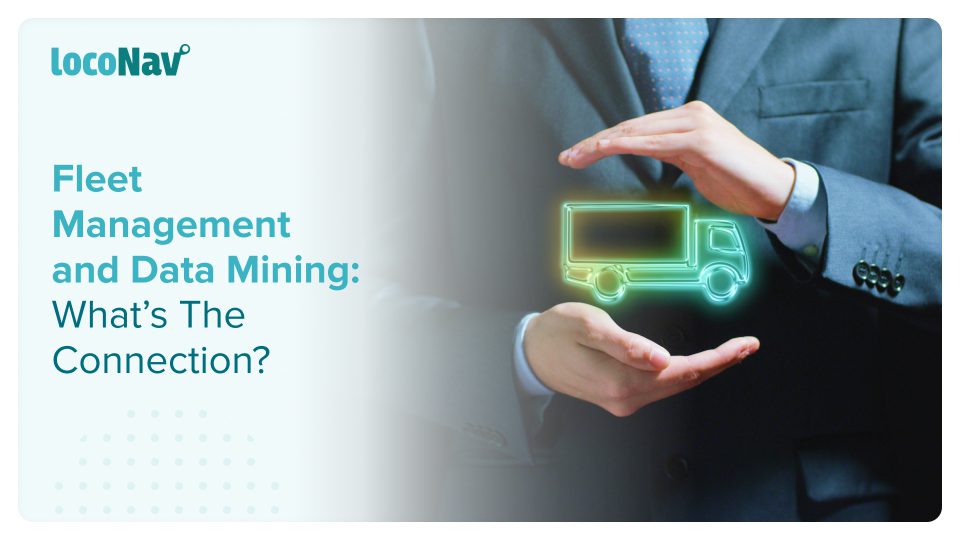
We are only now beginning to grasp the concept of big data. Adding to the confusion are data mining, machine vision, neural networks, and AI. What do these terms imply? And, more significantly, what do they have to do with the transportation industry?
Fleet management is one of the areas where data mining can provide significant benefits. Indeed, one of the most appealing aspects of telematics in the field is the potential to collect and analyse massive data sets, which might help businesses fine-tune their operations and boost safety for their drivers and fleet. But how exactly does data mining aid fleet management? This blog will assist you in decoding data mining in fleet management.
Manage your fleet efficiently with LocoNav’s Fleet Management Solutions!
What is Data Mining?
Data mining techniques are used to examine massive amounts of data (sometimes known as “big data”) in order to uncover patterns, trends, and connections. This activity frequently yields insights into how businesses might enhance their performance.
In data mining in fleet management, many fleets, for example, sift through data on near accidents to forecast and prevent future fatalities. Others mine location and time-of-day data to identify hot locations and redirect traffic to minimise delays or collisions at specific times of the day.
What are the Techniques of Data Mining?
There are 10 different types of data mining techniques. These are:
- Data Warehousing
Data warehousing is the practice of collecting and storing data before it is analysed.
- Association
Association is used to discover correlations or links between data points.
- Outlier Detection
Outlier detection is a critical component of keeping databases safe. Companies employ it to detect suspicious transactions, such as unusual credit card transactions that could indicate theft.
- Data Cleaning
Data cleaning entails structuring data, removing duplicate or faulty data, and trying to fill in any empty fields.
- Data Visualization
The conversion of data into graphic form to communicate its relevance to business stakeholders is known as data visualization.
- Machine Learning
Machine learning is the process through which computers learn on their own using algorithms.
- Clustering
Clustering is a technique for visually representing data, such as in graphs that demonstrate purchasing trends or sales demographics for a specific product.
- Neural Networks
Artificial neural networks try to digitally simulate the operation of the human brain. Neural networks employ a large number of computer processors (similar to how the brain uses neurons) to analyse data, make decisions, and learn in the same manner that humans do — or as close as feasible.
- Classification
Classification is the process of assigning data points from huge data sets to groups based on how they are used.
- Prediction
Predictive modelling attempts to convert data into a forecast of future action or behaviour. These models analyse data sets for patterns and trends before calculating the likelihood of a future result.
How Does Data Mining Link to Fleet Management?
Even the richest and most thorough data set would be useless if you couldn’t make sense of it. Much less if you don’t have access to all of the information you need to make sound decisions. To collect all data points, you need software like LocoNav’s Fleet Management System. Data Mining in fleet management will collect all of your fleet data, organise it, and present it to you in such a way that you can gain actionable insights from it.
Advantages of Data Mining in Fleet Management
Data mining in fleet management can do wonders. Let’s see how.
- Improved Driver Retention
Data mining in fleet management can be employed to retain your top drivers. Predictive modelling can assist you in analysing data that is already in your databases. This information could be utilised to learn more about your drivers and how they behave. If you have a problem with driver attrition, for example, you can look at the profiles of drivers who leave the organisation and discover what they have in common.
- Increased Customer Satisfaction
You might analyse your data to optimise your routes. A freight company can examine its customers’ data to have a better understanding of delivery and pickup expenses at each location. Knowing which clients and locations are more expensive allows you to cut loading and unloading times at these places, allowing you to enhance your route. Data mining in fleet management means better routes and subsequently, improved customer service.
- Advanced Driver Monitoring
Data mining in fleet management can be used by transportation businesses in two very distinct contexts. One is in operational management or needing to know if your drivers are following your specified routes or breaking traffic rules on a frequent basis.Â
Additionally, operational management may direct you to examine which of your drivers are going on longer stops than permitted, attendance and regularity rates, and delivery efficiency.
Then there’s the management of faulty conditions, such as detecting when your drivers exceed the speed limit or deviate from the route.
- Profitable Reselling
Another way data mining in fleet management can help a fleet manager is by determining how much to sell a vehicle for. If you want to sell some vehicles in your fleet, how do you determine the best-selling price? This may appear to be a simple task, but it is not.
Determining the residual value of your truck can be challenging, and an incorrect calculation can result in significant financial losses. Data mining allows you to leverage the vehicle’s age, performance, and condition to generate more precise calculations.
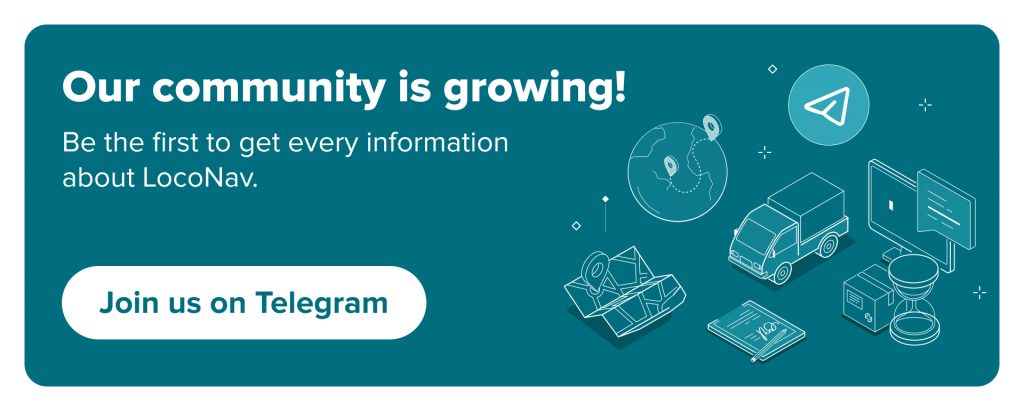